QMX-05 datasheet, cross reference, circuit and application notes in pdf format. Chess-Results.com is a powerful and dedicated server only for chess-results. The tournament archive of chess-results.com contains more than 40.000 tournaments from around the world.
- 177.0 5 8 联合王国的主体之一 苏维埃联盟: sov 129 3 161.43 534 392 32 6 44 82 193.2 133.0 67.1 543.4 353.3 1290.0 156 53 西班牙国: spr 26 3 24.75 0.96 151 78 7 4 16 27 1.6 53.0 66.7 134.1 30 48 瑞典: swe 7 3 6.14 40 29 3 3 9 15 3.4 56.0 186.9 35.0 281.3 12 25 瑞士: swi 2 3 4.07 25 14 4 7 11 52.8 52.8 4 唐努图瓦: tan 1 2.
- Volvo Penta Ford V8 5.0 5.8 Exhaust Manifold And Riser 38347 Starboad. From United States.
概率定义:
概率定义为一件事情发生的可能性,例如,随机抛硬币,正面朝上的概率。 Android apps on pc or maceffective curriculum ideas.
In addition, there are lots of ways to make money from your website besides just advertisements. I’m going to definitely show you how you can get advertisers to pay you money through display ad networks. However, I’ll also share lots of other unique ways to turn your website into a real business (i.e. 8 ways to profit from your website. Site flipping involves selling your website to an outside party in order to turn a profit. Many online resources exist for selling websites, including eBay’s page for website and business sales. Not all websites are suitable for flipping, and salability tends to depend on overall income.
联合概率:
包含多个条件,且所有条件同时成立的概率,记作:𝑃(𝐴,𝐵) 。
条件概率:
事件A在另外一个事件B已经发生条件下的发生概率,记作:𝑃(𝐴|𝐵) 。P(A1,A2|B) = P(A1|B)P(A2|B),需要注意的是:此条件概率的成立,是由于A1,A2相互独立的结果。
公式:
Mlt 0.5.8 For Mac Catalina
其中,w为给定文档的特征值(频数统计,预测文档提供),c为文档类别。
Mlt 0.5.8 For Mac Desktop
公式可以理解为:
其中c可以是不同类别。
公式分为三个部分:
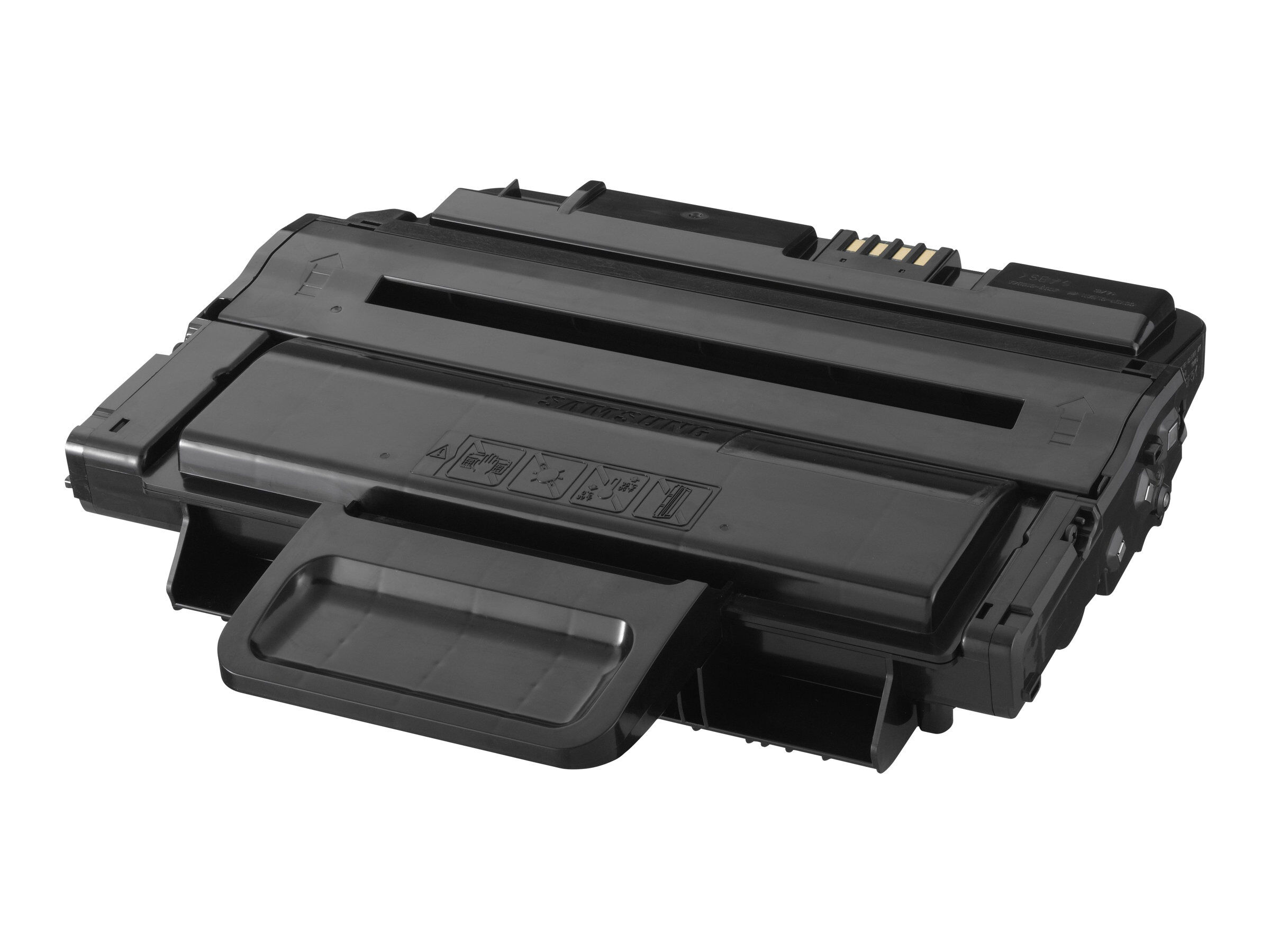
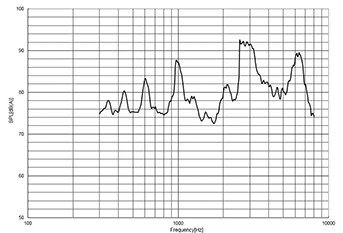
𝑃(𝐶):每个文档类别的概率(某文档类别词数/总文档词数)
𝑃(𝑊│𝐶):给定类别下特征(被预测文档中出现的词)的概率
计算方法:𝑃(𝐹1│𝐶)=𝑁𝑖/𝑁 (训练文档中去计算)
𝑁𝑖为该𝐹1词在C类别所有文档中出现的次数
N为所属类别C下的文档所有词出现的次数和
𝑃(𝐹1,𝐹2,…): 预测文档中每个词的概率
举个栗子:
现有一篇被预测文档:出现了都江宴,武汉,武松,计算属于历史,地理的类别概率?
Mlt 0.5.8 For Mac Catalina
历史:𝑃(都江宴,武汉,武松│历史)∗P(历史)=(10/108)∗(22/108)∗(65/108)∗(108/235) =0.00563435
地理:𝑃(都江宴,武汉,武松│地理)∗P(地理)=(58/127)∗(17/127)∗(0/127)∗(127/235)=0
拉普拉斯平滑:
思考:属于某个类别为0,合适吗?
从上面的例子我们得到地理概率为0,这是不合理的,如果词频列表里面有很多出现次数都为0,很可能计算结果都为零。
解决方法:拉普拉斯平滑系数。

sklearn朴素贝叶斯实现API:
案例:新闻分类
优点:
Mlt 0.5.8 For Mac Os
- 朴素贝叶斯模型发源于古典数学理论,有稳定的分类效率。
- 对缺失数据不太敏感,算法也比较简单,常用于文本分类。
- 分类准确度高,速度快
缺点:
- 需要知道先验概率P(F1,F2,…|C),因此在某些时候会由于假设的先验模型的原因导致预测效果不佳。
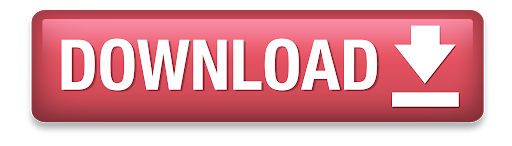